Confirmation Bias: Social Media to Users' Beliefs
- Lawrence Chan
- Dec 31, 2018
- 13 min read
Updated: Jan 1, 2022
Presentation: https://docs.google.com/presentation/d/1vo2NhMUm2CCR_FwpbNVG6dS0He2qYeTZ73QWsKDOLvs/edit?usp=sharing
Abstract
This report will discuss the effect of social media on encouraging and reinforcing confirmation bias in the user’s existing beliefs. We will study the case of users’ beliefs in the context of climate changes (global warming) to demonstrate the social impacts on society, caused by the role of social media in reinforcing confirmation bias. The report will first identify the issue through primary and secondary data regarding the impacts on society. Furthermore, the causes of social media content distributing biased content to users will be analyzed. Moreover, the report will evaluate existing solutions and recommend the use of sentiment analysis algorithm, with detailed implementation of this method to incentivise all stakeholders to adopt this system.
Introduction
Since the conception of social media platforms ten years ago, they have evolved from being a communication tool to a source of news and information. According to a survey conducted by US-based think tank Pew Research Center, 68% out of 4581 respondents claimed they acquire news from social media (Matsa et al., 2018). The phenomenon by itself is indifferent. However, the concern arises when these platforms tailor information to match user preferences. This implies that news seen by users have the tendency to skew towards a particular stance for a given issue. Whilst biased information is arguably harmless for less important topics such as entertainment, information about serious issues such as politics, and global warming would have a tremendous impact on society. In this report, global warming is used as an example to illustrate the concern.
First of all, we would like to assert the validity of global warming. 97% of publishing climate scientists have reached a consensus that global warming is caused by humans (Cook, 2016). Furthermore, according to NASA, it is clear that the atmospheric carbon dioxide concentration has almost doubled since the Industrial Revolution (NASA, 2018). This correlates with the data showing a global temperature rise of nearly 2 degrees Celsius.
Though given the abundance of supporting scientific evidence, there exists many global warming deniers and skeptics. From Youtube, we searched for a global warming denying video (Figure 1) and found a top rated comment with 92 likes (which can be viewed as affirmation from other users), claiming that “global warming and cooling has existed since the beginning of creation and it is arrogant to believe that human is powerful enough to change and control weather on a global scale.” (Figure 2). Moreover, Gallup, a US management consulting company has conducted a survey on environmental related topics in 2018 in the US. They found that 19% of respondents are skeptical towards global warming (Brenan et al., 2018). In short, given the plethora of evidence, there is still a large group of people who do not believe that global warming is caused by humans and its detrimental impact on the future of mankind.

Having such a stance may seem inoffensive, but it can cause serious damages. In the case of fossil fuels lobbying, where fossil fuels companies pay huge money as high as US$100M to political candidates in an attempt to influence governmental policy that favours them (Center for Responsive Politics, 2018) (Figure 3). Recall that 19% of US citizens are skeptical when it comes to global warming, it is much higher when looking at US Republicans, in which 69% of them denied the existence of global warming (Popovich et al., 2017). Consequently, according to data from the US Federal Election Commission, a significant portion of lobbying money from oil companies goes to Republican candidates (Center for Responsive Politics, 2018) (Figure 4). As giant corporates take advantages of the confirmation bias Republicans have on denying global warming, not only that more money is invested in factories that would aggravate global warming, their confirmation bias gave the Republicans a sense of justification. We can clearly see that merely having a stance can lead to irreversible damages, in this case, damages to the environment. If those 69% of US Republicans are able to overcome their confirmation bias and actually research disconfirming but scientific evidence, the scenario would perhaps be much better.

By looking at the primary and secondary data related to global warming and the confirmation bias behaviours of users compiled above, we illustrated how social media can enhance confirmation bias and the seriousness of failing to analyze the big picture of such a serious issue. Next, we will discuss the causes of why social media contributes to the confirmation bias of the users.
Causes
The behavior of social media users falling into the traps of confirmation bias on certain issues is greatly accelerated by the algorithm social media implement on their platform. Social media tend to display biased information in order to appeal to individual users, encouraging them to continue consuming their contents and staying on their platform. There are two major algorithms that social media usually adopt to achieve this purpose: collaborative filtering and association rule mining.
i. Collaborative filtering Collaborative filtering is a recommendation algorithm based on the assumption that the best content recommendations for a person are derived from the surrounding people who share similar tastes or preferences. This algorithm can be found on Amazon, Facebook, Netflix, etc. (Grover, 2017). Before this algorithm recommends an item to a person, it would find all individuals who share similar preferences with that person. For example, Person B is similar to Person A since he likes the same items Person A likes as well. Then, the algorithm will generate a list of items that those group of people prefer, and thus recommend them to Person A.
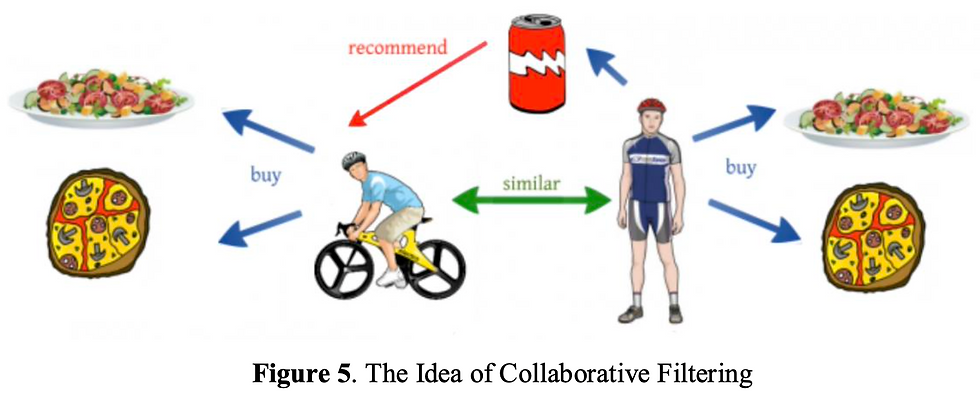
The ultimate result of collaborative filtering is called echo chamber. Echo chamber refers to a situation in which beliefs are amplified or reinforced by communication and repetition inside a closed system (Jameel, 2016). Given that many social media users are surrounded by like-minded people such as their friends, their recommended contents which are generated from their friends are more likely to support their own opinion, thus reinforcing their existed beliefs. Conversely, the contents that disprove their existing views would not be shown to them, and so their existing belief would not be challenged and would be polarized.
ii. Association Rule Mining Association Rule Mining is a machine learning algorithm for discovering relations between variables in large databases. With sufficiently large volume of behavioral data, the algorithm can observe frequently occurring patterns, correlations, or associations from datasets (Rai, 2018). In social media, this algorithm can be found in the recommendation by history, especially on YouTube. It recalls the users’ history of content consumption and analyze the relationship between each content, then recommend the content sharing similar relations with those histories.
To demonstrate this algorithm on YouTube, we conducted a simple experiment. We access YouTube with a new account, then watched 10 videos denying climate change, with one video of video game streaming as a reference point. As the result shown in Figure 6, after a large enough record of video history is created, 9 out of 18 videos recommended are related to climate change denial, 8 of them related to other social or climate issue, and 1 of them about video game streaming. The close relation can be found between recommended videos and consumed videos, proving that association rule mining is implemented in YouTube.
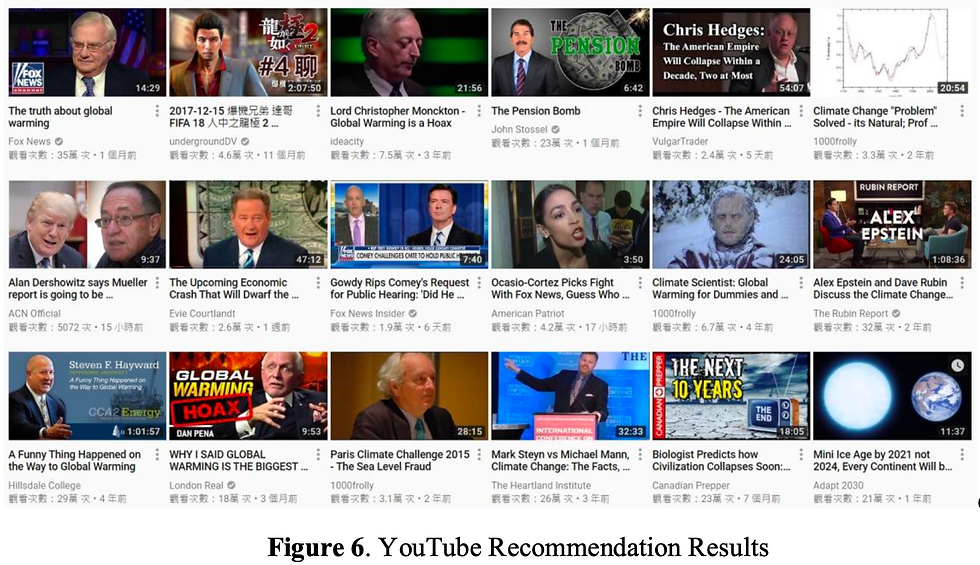
The consequence of association rule mining can be known as filter bubble. It is an effect proposed by Eli Pariser, suggesting that the selective and personalized information given by social media or website will eventually make us closed-minded, less intellectually adventurous, and more vulnerable to propaganda and manipulation (Weisberg, 2011). With the previous experiment in mind, if a user has a strong interest in denying climate change, the association rule mining algorithm would recommend videos that favor his belief of climate change denial, and unlikely to show videos that prove climate change is correct to challenge his existing belief. As a result, their existing beliefs would be strengthened or even polarized.
The primary motive behind these aforementioned algorithms is because service providers want to fulfill what users desire to see, resulting in confirmation bias. Confirmation bias makes people look for information that is consistent with what they think, leading them to avoid or forget information that requires them to change their minds. People often exhibit a strong preference for their existing mental model about climate change, making them susceptible to confirmation bias and thereby misinterpret scientific data. People weigh up the costs of their wrong beliefs, rather than investigating the truths in a neutral, scientific way.
Let us consider the case of Canada. From Figure 7, although they are less likely to be affected by climate change, they have a higher level of awareness of global warming. Also, we found out that people within the same geological area will perform similar searches. So, search engine makes use of popular search results inputted by Canadian citizens and aggregate similar related information to other Canadians. Therefore, Canadian browsers generate supportive data, such as rising of sea level and continuous retreat of sea ice, that further reinforce their beliefs and stance on global warming, as evident from Figure 8.
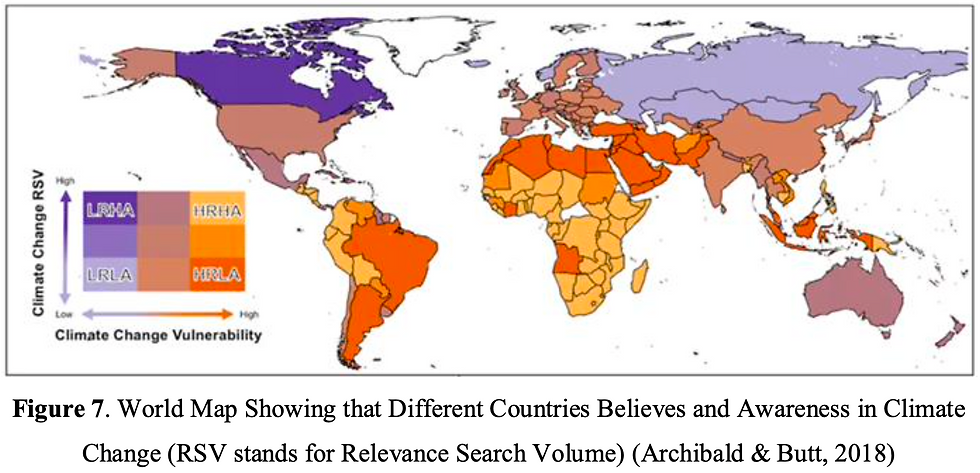
From Figure 8, we could see the top search results are climate change facts, news, warning etc. This proves that a lot of Canadians believe in global warming, so these people will have a biased search on some supportive data like rising of sea level, continue retreat of sea ice. All these data help to reinforce their current beliefs.
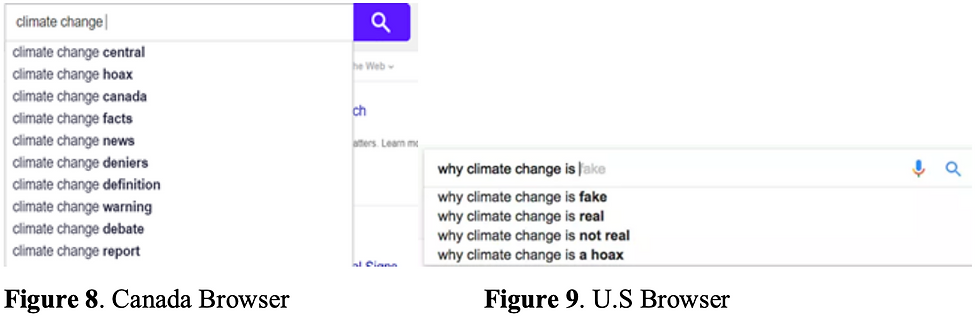
Surprisingly, from the map shown in Figure 7, the American relevance search volume (RSV) in global warming is lower, compared to other well-developed countries. This can be attributed back to the general behaviour of U.S. citizens. Most Americans do not believe in global warming, thinking that this issue is fake. As a result, the most common search results are climate change is fake, not real or a hoax as shown in Figure 9. This will intensify the effect of confirmation bias because when users search for information, US search engine only presents supporting evidence such as Antarctic sea ice is growing at the fastest rate ever, or the rise of global temperature is only due to the natural fluctuations in temperature that happens in every several centuries as part of a natural climate cycle. These people are unable to investigate climate change in a scientific way as opposing scientific facts that are related to the rise of sea level, retreat of glaciers, and sea ice are not displayed under these searches. All these biased search results will only reinforce users in believing in their current ideology.
From these two cases, users of the internet and social media are more likely to search for information that confirms their preexisting beliefs, forming a vicious cycle (Figure 10).
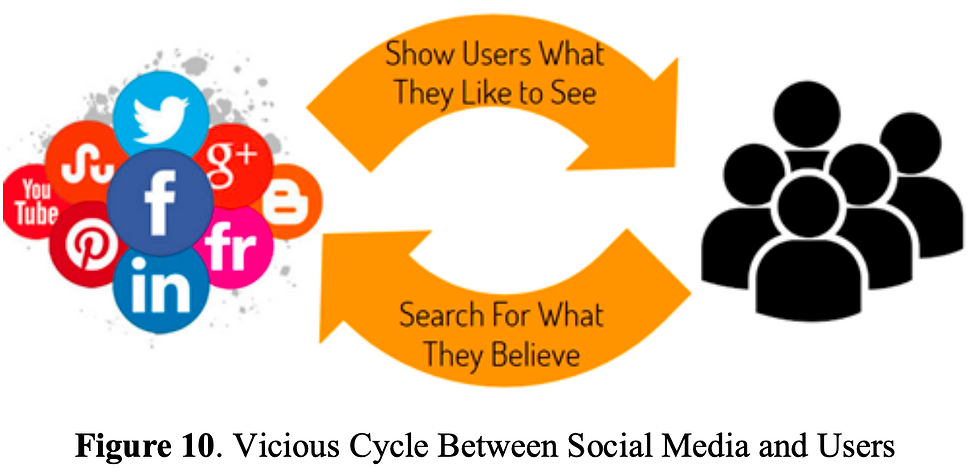
Existing Solutions
There are several existing solutions to reduce confirmation bias among users in social media. In the following, we will further evaluate the drawbacks of those methods in the context of climate change.
First, Human Fact Checking is one of the direct methods to solve the problem. The mechanism behind this method is straightforward. Several professionals analyze the content to determine the credibility of the article and then label remarks on the writings to warn the readers about their trustworthiness (Wells, 2018). However, this collaboration between Facebook and Factcheck.org has left a lot to be desired. For each article, they need at least 4 editors, and 2 fact-checking organizations to reach an agreement, and thus only an average of less than 1 fake news debunked each day (Wells, 2018). We believe that this approach is very inefficient, as it is time-lagging and thus the damage has been done already.
Not only so, but it also introduces a new dimension of human biases with the addition of experts. They may have a certain preference on a particular issue and would exert their influences to publish what they think is right. Their behaviour does not encourage neutrality of information in social media and would, in turn, create more confirmation bias among social media users.
Second, Tumblr has also created a random mode on their page to solve the confirmation bias. Users can choose whether their recommendation posts will be considered from their search history or not (Figure 11). However, this random mode is not the default, so if users do not consider with an unbiased attitude, they will not choose to implement the random mode. Therefore, this strategy is not effective for the majority of the user base.
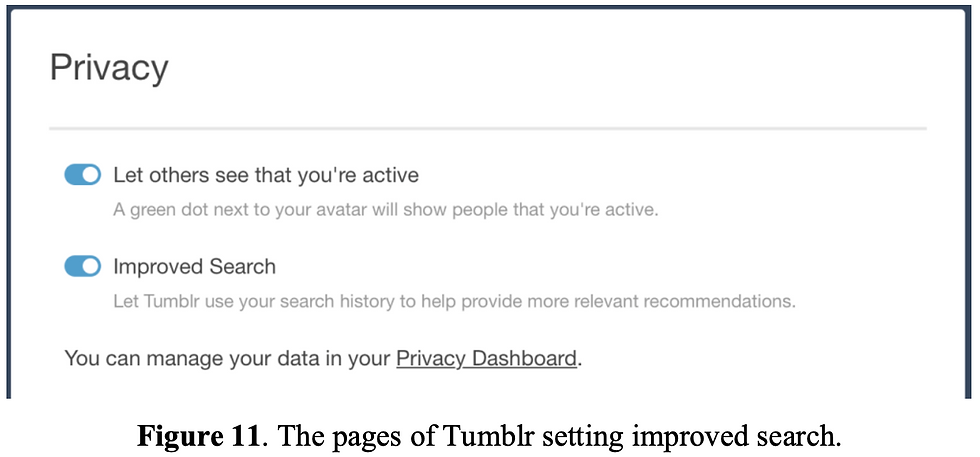
In the case of climate change, our ideal information sorting would generate an equal proportion of news supporting climate change, news denying climate change, and news that have a neutral stance on global warming. However, random selection does not guarantee this ideal outcome. Such a method will result in producing an unequal proportion of news where either all are supporting the climate change or all denying the climate change. Furthermore, users often only use this option to find what they like and search for more corresponding information. As a result, random search does not help solve the issue of the enhancement of confirmation bias. Instead, it is more preferable to provide the users with diverse objective facts, allowing them to make well-informed judgments.
Lastly, there are existing scam-checking websites by the collaboration of Google and Facebook, they are using an algorithm to analyze news portal or websites, based on domain location, the age of the website, and its popularity (Wendling, 2018). However, since the algorithm does not check the content due to the complicated nature of human language, it does not provide a fair judgment to the websites. Consider using the above algorithm in solving misinformation concerning climate change. Even if a legit news portal is set up, the algorithm would think that it is still new and not popular, thus labeling it as a scam in the end. This does not solve confirmation bias at its core.
Nevertheless, we do think that sorting information with algorithms would be the best choice to avoid bias caused by the human manipulation of data.
Recommendation
To reduce confirmation bias caused by social media, we suggest replacing the current association algorithm with sentiment categorization in order to present to the users all sides of information regarding each issue. Even if the users are not interested in learning more about the opposing views and opinions, their awareness will be increased and their beliefs will be expanded due to the mere-exposure effect. This algorithm greatly benefits both social media platforms and users, leading to a well-informed and knowledgeable society.
Our recommended algorithm makes use of sentiment analysis in order to ensure users are presented with a wide range of unique views on every topic. Sentiment analysis is an artificial intelligence technology that determines people’s opinions toward different ideas (“What is Sentiment Analysis?”, n.d.). By analyzing the words that are used through natural language processing (NLP), it is able to distinguish the contextual polarity within the information. This means that the analysis can distinguish whether the piece of writing is supporting, arguing against, or neutral towards a certain topic. The integration of sentiment analysis is demonstrated in Figure 12. All information must be converted into text-based formats for further analysis. Video clips will undergo the process of subtitles auto-generation to produce transcripts of the speech, while texts will be extracted from articles, posts, and blogs. These text documents will then be categorized into current existing topics through topics extraction, which is a machine learning model that makes use of statistical techniques in grouping words based on their topic similarities (“What is Topic Modeling?”, 2017). The most important topics are then selected based on their search popularity on the Internet. For each topic, sentiment analysis is used to further classify them within the spectrum from strongly against to firmly supporting. With this new system in place, it is able to identify each user’s stance on each topic by analyzing their behaviors. When distributing information to users, the system will balance the content by putting a heavier emphasis on the opposing views of the user in order to enhance the exposure of multiple angles of the topic.
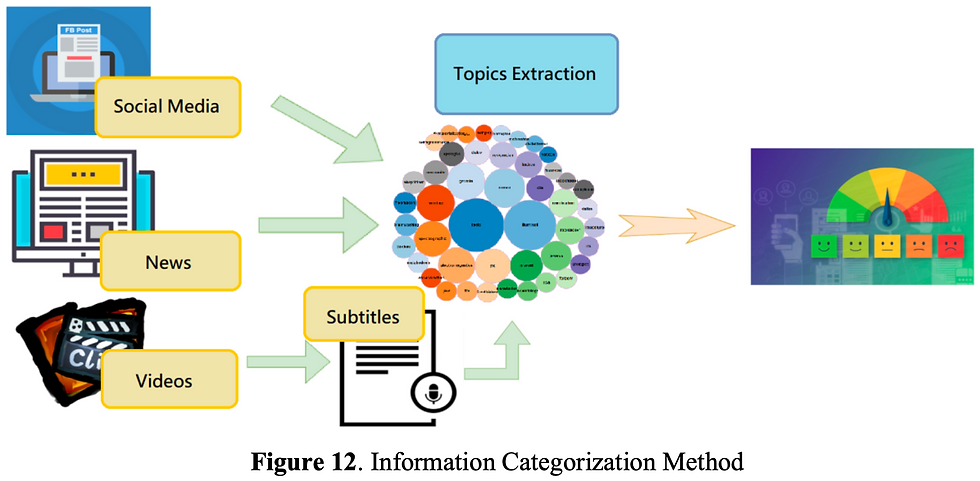
Even if users decide to ignore all the content that differs from their beliefs, their attitudes toward these social issues will change over time due to the mere-exposure effect. The mere-exposure effect is “a psychological tendency that causes individuals to prefer an option that they have been exposed to before to an option they have never encountered, even if the exposure to the first option was brief” (“What is Mere Exposure Effect?”, n.d.). Because of this effect, repeated prior exposure to an opinion can lead to a more positive attitude towards it. This allows different views to be more acceptable to users, making them more open-minded towards these views. The mere-exposure effect is dependent on the content’s cognitive fluency, which is the ease of processing information (O’Connor, 2017). This is because humans tend to accept or believe something that is easy to comprehend. Since social media is heavily driven by visuals, the content is very attractive and simple to process. Repeated exposure to opposing opinions also simplifies the processing effort over time. As a result, even if the users do not interact with the opposing beliefs, their attitudes towards them will be changed over time as they become more familiar with these views.
To implement this solution, we need to convince our stakeholders to adopt our recommendation. Making use of social media’s loss aversion attitude, we would convince them with the detrimental consequences fake news have on their platform. Failure to regulate content and police their site would lead to a decline in monthly active user base. This has already caused an evaporation of $120B in Facebook’s market value and a 20% fall in Twitter’s share price over 2018 (Stewart, 2018). To convince our users, we have to adapt user behaviour and make use of their decision avoidance. We would implement our solution as the default option while also allowing the users to consciously make the decision to switch it off. However, with regret aversion exhibited by users, they would most likely stick with the status-quo option, thus adopting our recommendation. When all stakeholders agree to break this vicious cycle of misinformation, social media will be able to build an intelligent user base, with lesser fake news being echoed by the users.
Conclusion
In conclusion, our solution does not censor any information. Instead, our algorithm displays a wide array of diverse information, both confirming and disconfirming evidence to the users. With the help of the mere-exposure effect, our users are able to evaluate both sides of the story more effectively and form a holistic opinion towards social issues. In the long run, we want to create a well-informed society with the ability to investigate both sides of the topic, thus overcoming confirmation bias. This would be a win-win outcome for all parties.
References
Archibald, C., & Butt, N. (2018). Google searches reveal where people are most concerned about climate change. Retrieved from https://theconversation.com/google-searches-reveal-where-people-are-most-concerned-about-climate-change-102907
Brenan, M., & Saad, L. (2018, March 28). Global Warming Concern Steady Despite Some Partisan Shifts. Gallup. Retrieved from https://news.gallup.com/poll/231530/global-warming-concern-steady-despite-partisan-shifts.aspx
Center for Responsive Politics. Oil & Gas. (2018). Retrieved from https://www.opensecrets.org/industries/indus.php?cycle=2018&ind=E01
Cook, J., Oreskes, N., Doran, P. T., Anderegg, W. R., Verheggen, B., Maibach, E. W., . . . Rice, K. (2016). Consensus on consensus: A synthesis of consensus estimates on human-caused global warming. Environmental Research Letters, 11(4), 048002. doi:10.1088/1748-9326/11/4/048002
Georgia Wells, Lukas I. Alpert. In facebook’s effort to fight fake news, human fact-checkers struggle to keep up. Oct 18, 2018. Available from: https://www.wsj.com/articles/in-facebooks-effort-to-fight-fake-news-human-fact-checkers-play-a-supporting-role-1539856800.
Grover, P. (2017). Various Implementations of Collaborative Filtering – Towards Data Science. Retrieved fromhttps://towardsdatascience.com/various-implementations-of-collaborative-filtering-100385c6dfe0
Jameel, S. (2016). Infographic: The Echo Chamber Effect. Retrieved fromhttps://zestythings.com/infographic-the-echo-chamber-effect/
Matsa, K. E., & Shearer, E. (2018, September 21). News Use Across Social Media Platforms 2018. Pew Research Center. Retrieved from http://www.journalism.org/2018/09/10/news-use-across-social-media-platforms-2018/
Mike Wendling. Solutions that can stop fake news spreading. 30 January 2017. Available from: https://www.bbc.com/news/blogs-trending-38769996.
NASA (2018). Climate change evidence: How do we know?. Retrieved from https://climate.nasa.gov/evidence/
Ponsot, E. (2017). "Why climate change is fake"-What Americans google about global warming and why it matters. Retrieved from https://qz.com/995262/why-climate-change-is-fake-what-americans-google-about-climate-change-and-why-it-matters/
Popovich, N., & Albeck-ripka, L. (2017, December 14). How Republicans Think About Climate Change - in Maps. Retrieved from https://www.nytimes.com/interactive/2017/12/14/climate/republicans-global-warming-maps.html
Stewart, E. (2018, July 28). The $120-billion reason we can't expect Facebook to police itself. Retrieved from https://www.vox.com/business-and-finance/2018/7/28/17625218/facebook-stock-price-twitter-earnings
Rai, A. (2018). Association Rule Mining: An Overview and its Applications. Retrieved from https://www.upgrad.com/blog/association-rule-mining-an-overview-and-its-applications/
Weisberg, J. (2011). Eli Pariser's The Filter Bubble: Is Web personalization turning us into solipsistic twits? Retrieved fromhttps://slate.com/news-and-politics/2011/06/eli-pariser-s-the-filter-bubble-is-web-personalization-turning-us-into-solipsistic-twits.html
Archibald, C., & Butt, N. (2018). Google searches reveal where people are most concerned about climate change. Retrieved from https://phys.org/news/2018-09-google-reveal-people-climate.html
What is Sentiment Analysis? - Definition from Techopedia. (n.d.). Retrieved from https://www.techopedia.com/definition/29695/sentiment-analysis
What is Topic Modeling?. (2017). Retrieved from https://provalisresearch.com/blog/topic-modeling/
What is mere exposure effect? definition and meaning. (n.d.). Retrieved from http://www.businessdictionary.com/definition/mere-exposure-effect.html
O’Connor, K. (2017). Attitudes Exposed: How Repeated Exposure Leads to Attraction | Social Psych Online. Retrieved from http://socialpsychonline.com/2017/01/attraction-psychology-mere-exposure/
Comments